Optimise and detox your YouTube Ad campaigns using n-gram analysis
Reduce tenfold the time sunk into the optimisation process
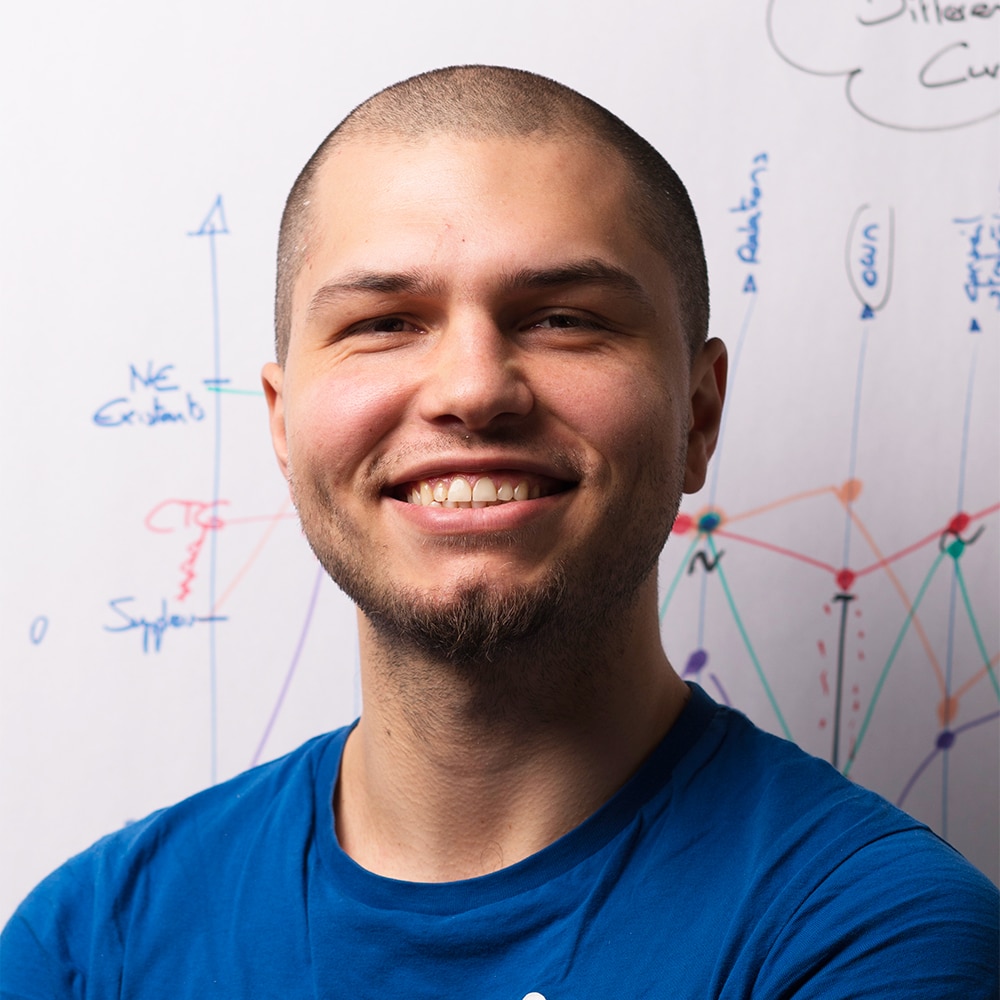
By ARBEN KQIKU
YouTube Ad campaigns and the toxic channel problem
Youtube is more than just a video-sharing website. Considered separately from the Google ecosystem to which it belongs, it is the world’s second largest search engine (or, depending on how you crunch the numbers, the third largest). It won’t come as news to any marketing professional that this represents a huge opportunity for ad campaigns. One of the big problems encountered in YouTube campaigns above a certain size is the presence of toxic channels that are entirely irrelevant to you or your client’s goals. Google Ads allows you to follow in detail the channels where your ads have appeared, but how to efficiently manage an Ads account with multiple campaigns running ads that simultaneously appear on thousands of channels? Viewed individually, such toxic YouTube channels are easy to shrug off as part of your campaign’s error margin. After all, how bad can it be to have a few misdirected impressions here and there? You have bigger fish to fry—namely, the YouTube channels getting the bulk of the impressions which are the real objective of your campaigns. However, if you sum up all of these irrelevant channels that you have been ignoring due to prioritization of your resources, you may find it adds up to more wasted spend than you imagine.Solving the toxic channel problem at scale: n-grams to the rescue
Even if you identify the toxic YouTube channel problem, the prospect of fixing it can be daunting. Exclusion of irrelevant and undesired channels can only be done at the campaign level. This means that, unless you can figure out a better approach, you would need to manually remove each and every toxic channel independently. Let’s put this problem in context at a relatively modest scale. Imagine, for example, running five YouTube campaigns across ten different Google Ads accounts. This means repeating the same tedious and costly operation fifty times. The time wasted on such measures quickly gets out of hand. Unchecked, the spending waste is likely much higher than you would like. We have a solution: use an n-gram analysis! An n-gram is an aggregation or collection of symbols or words often used in natural language processing, it enables machine learning algorithms to understand, and/or predict the probability of, words (or grams), based on the context they are used in. Replace the n value with the number of words in a given grouping that you want to identify, and voilà—n-grams. In the context of a YouTube ad campaign, performing an n-gram analysis will allow you to reverse-engineer your data to further optimise your impressions, use of keywords, and ad spending. In the next and final part of this article, we will take a look at a practical example of how to run an n-gram analysis on a fictitious ad campaign.Putting n-grams to work in your YouTube campaigns
Step one: export the information from each of your different accounts about all channels where your ads were displayed.
In the following table, you can see a small sample of channels that have generated just a single impression. All of these channels fall outside of our targeted audience and are a source of wasted spend. We want to exclude them, but to do so individually would be excruciating.
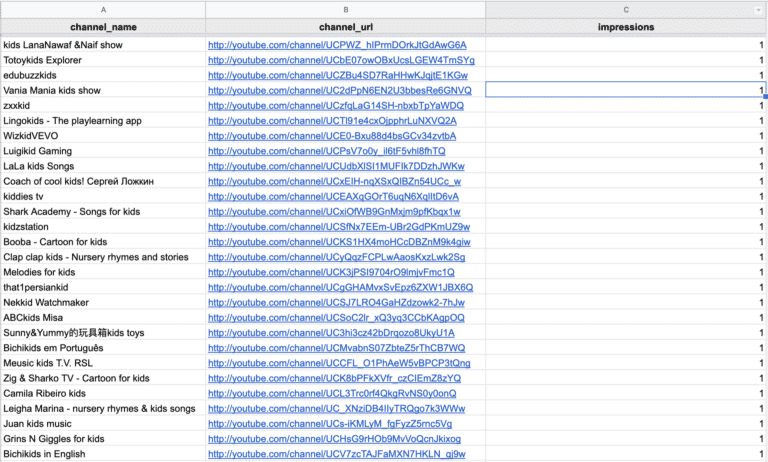
Step two: run an n-gram analysis to associate impressions with keywords from channel titles.
In our current example, we have decided to look at unigrams (or 1-grams).
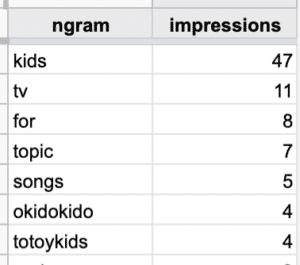
Step three: analyze the n-grams you have chosen and assess whether or not they are relevant to your goals.
In the fictitious example we’re looking at, we find that channels containing the unigram “kids” have generated 47 undesired impressions. Bear in mind that in the context of real campaigns, these numbers are likely to be much higher, often upwards of 10’000 impressions or more.
Step four: filter out and automate the exclusion of the n-grams that you decide are toxic to your YouTube campaigns.
In this example, this will mean excluding all 47 channels that contain the unigram “kids” which have been capturing large numbers of impressions but do not fall within our target audience. To do this, go back to the tab with the raw data, filter out all the channel names that contain the unigram “kids”, and exclude them in bulk!
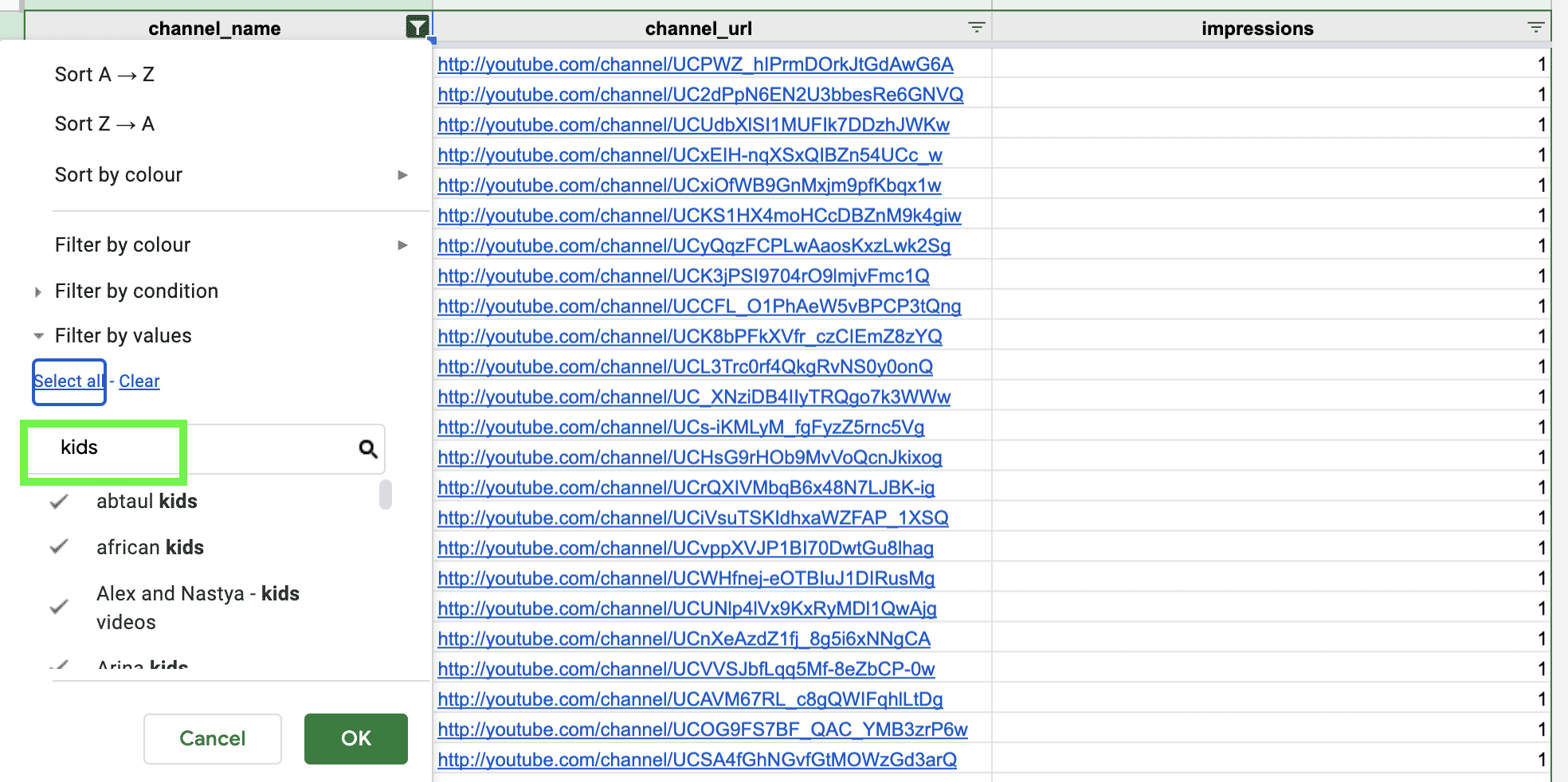
The simplicity of such an n-gram analysis is deceptive. At scale, this represents a truly powerful tool to help you break down your data, optimise your impressions, and efficiently manage your YouTube campaigns.
Time for a detox?
At comtogether, we automate everything except the part where we export the data and decide which n-grams are toxic for our clients’ goals. The n-gram analysis allows us to reduce tenfold the time sunk into the optimisation process of a Google Ads account. A job that would otherwise take fifty+ hours takes us less than five.
This is just one of many creative methods that we use to optimise our workflow and guarantee optimal results for our clients.
If you have any questions on the nitty-gritty of YouTube campaigns, toxic channels, n-gram analysis, or anything else related to the world of online marketing, don’t hesitate to get in touch. We are here to help.
About the author
You want to learn more about Google Ads ? Discover our Whitepaper ! 👇